The value of data is rising exponentially. The cloud has fundamentally altered the way we manage and store that data. Cloud computing is easy. Efficient. Scalable. Cost-effective. To leverage these capabilities to their full potential, you need tools designed for the modern computing landscape, built to handle unprecedented challenges and deliver extraordinary insights.

Role of elt/etl in the modern analytics architecture
Many organizations we engage with are seriously considering transforming their business and moving some (or all) of their IT operations into the cloud. A lot of executives I have encountered are struggling with the same question: “How do I get started?” There is a strong case to be made that starting with your Enterprise Data Warehouse (EDW), or at least a data mart, is the fastest, and most risk-free path, with added upside potential to increase revenue and set you up for future growth.
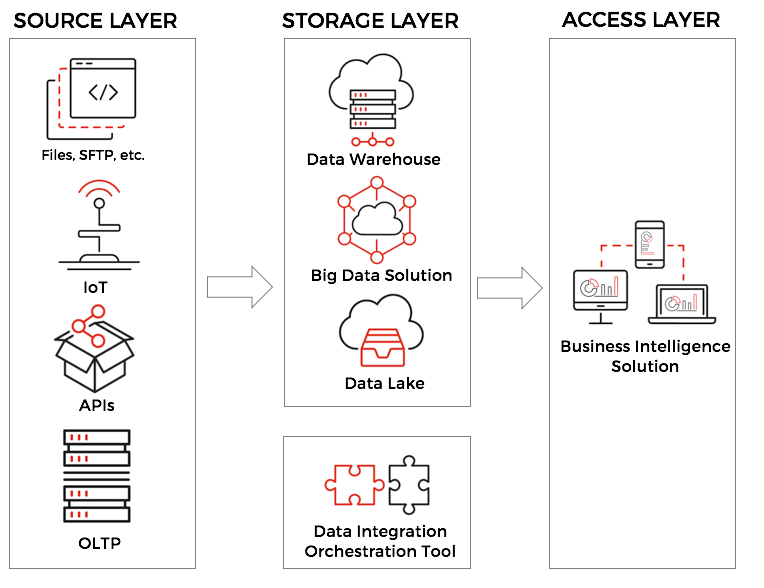
Etl vs elt: understanding the difference
The primary differences between ETL and ELT are how much data is retained in data warehouses and where data is transformed.
With ETL, the transformation of data is done before it is loaded into a data warehouse. This enables analysts and business users to get the data they need faster, without building complex transformations or persistent tables in their business intelligence tools.
Using the ELT approach, data is loaded into the warehouse or data lake as is, with no transformation before loading. This makes jobs easier to configure because it only requires an origin and a destination.
The ETL and ELT approaches to data integration differ in several key ways.
- Load time — It takes significantly longer to get data from source systems to the target system with ETL.
Transformation time — ELT performs data transformation on-demand, using the target system’s computing power, reducing wait times for transformation. - Complexity — ETL tools typically have an easy-to-use GUI that simplifies the process. ELT requires in-depth knowledge of BI tools, masses of raw data, and a database that can transform it effectively.
- Data warehouse support — ETL is a better fit for legacy on-premise data warehouses and structured data. ELT is designed for the scalability of the cloud.
- Maintenance — ETL requires significant maintenance for updating data in the data warehouse. With ELT, data is always available in near real-time.Both ETL and ELT processes have their place in today’s competitive landscape, and understanding a business’ unique needs and strategies is key to determining which process will deliver the best results.
Benefits of elt
The explosion in the types and volume of data that businesses must process can put a strain on traditional data warehouses. Using an ETL process to manage millions of records in these new formats can be time-consuming and costly. ELT offers a number of advantages, including:
- Simplifying management — ELT separates the loading and transformation tasks, minimizing the interdependencies between these processes, lowering risk, and streamlining project management.
- Future-proofed data sets — ELT implementations can be used directly for data warehousing systems, but oftentimes ELT is used in the data lake approach in which data is collected from a range of sources. This, combined with the separation of the transformation process, makes it easier to make future changes to the warehouse structure.
- Leveraging the latest technologies — ELT solutions harness the power of new technologies in order to push improvements, security, and compliance across the enterprise. ELT also leverages the native capabilities of modern cloud data warehouses and big data processing frameworks.
- Lowering costs — Like most cloud services, cloud-based ELT can result in the lower total cost of ownership, because an upfront investment in hardware is often unnecessary.
Flexibility — The ELT process is adaptable and flexible, so it’s suitable for a variety of businesses, applications, and goals. - Scalability — The scalability of a cloud infrastructure and hosted services like integration platform-as-a-service (iPaaS) and software-as-a-service (SaaS) give organizations the ability to expand resources on the fly. They add the compute time and storage space necessary for even massive data transformation tasks.Although it is still evolving, ELT offers the promise of unlimited access to data, less development time, and significant cost savings. In these and other ways, the cloud is redefining data integration.